Machine Learning: Revolutionary Tool or Illusion of Mastery?
Is Machine Learning an essential strategic lever or a cognitive trap reinforcing the biases of its users? Learn how manufacturers and tech companies can unlock its full potential without falling into the illusion of neutrality and full automation.
MANAGEMENT
Lydie GOYENETCHE
2/2/20255 min read
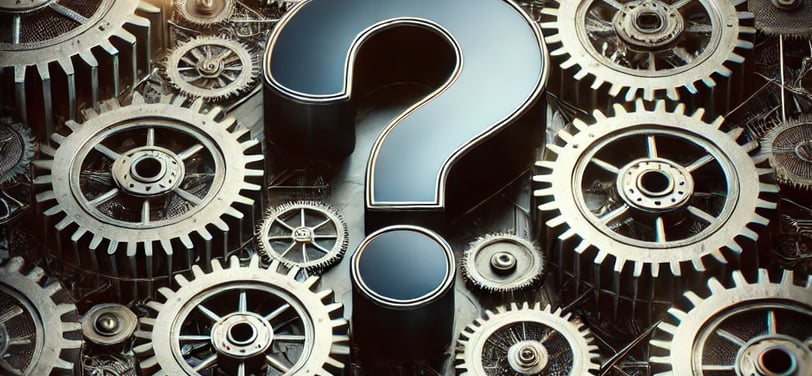
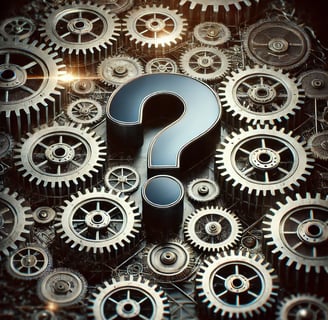
Machine Learning: Opportunities, Limitations and Applications in Business
Machine learning is now a key component of artificial intelligence and a powerful lever for automating business processes. It allows you to analyze large amounts of data, identify trends, and make decisions based on predictive models. However, while machine learning offers undeniable advantages, it also has limitations, especially when it comes to human decisions influenced by emotional factors or complex contexts.
In this article, we'll explore the principles of machine learning and how it works, the opportunities and challenges it presents, its application in marketing, communication, and business management, and its use in purchasing and inventory management.
Understanding Machine Learning
Machine learning is a branch of artificial intelligence that allows machines to learn from data, without explicit programming. It is based on algorithms that can detect patterns and make predictions based on past information.
There are several types of Machine Learning. Supervised learning relies on models trained on labeled data, such as classifying emails as spam or non-spam. Unsupervised learning allows models to analyze data without labels and detect groupings, such as customer segmentation in marketing. Reinforcement learning is based on a trial-and-error mechanism, as can be seen in video games or self-driving cars.
The main players in machine learning are tech giants such as Google, Amazon, Microsoft, and IBM. These companies offer specialized platforms that make it easy for businesses to train and deploy models.
Benefits and challenges of machine learning
Machine learning offers several benefits to companies. It enables the automation of repetitive tasks, accurate predictions based on big data analysis, business process optimization, and increased personalization of the customer experience through targeted recommendations.
However, there are also challenges to using it. Machine learning is entirely dependent on data and cannot function effectively without quality information. It is also subject to algorithmic biases that can reproduce discriminations present in training data. Some decisions made by these algorithms are difficult to interpret, especially in the banking sector where it is sometimes complicated to understand how a loan is allocated. Finally, it shows its limits when it comes to integrating complex human elements, such as emotions and intuition.
Machine Learning in Marketing and Sales Management
Machine learning is often touted as a revolutionary tool for marketing and sales management. However, its effectiveness depends strongly on the context of application.
In the field of marketing, it allows for fine customer segmentation and the optimization of advertising campaigns. Streaming platforms and e-commerce sites also use these templates to offer personalized recommendations to users. Chatbots and virtual assistants improve the customer experience by automating answers to common questions.
However, these advances have their limits. Human decisions are influenced by unpredictable factors such as mood, economic context, or social trends. Sentiment analysis, while effective in some areas, remains imperfect and struggles to capture irony, emotion, or ambiguity in customer feedback. In addition, business intuition and the perception of weak signals remain elements that are difficult to replace by an algorithm, which limits the effectiveness of machine learning in strategic business management.
Machine Learning and Procurement Management
One of the major challenges of Machine Learning in industrial and commercial companies is inventory and purchasing management. It is used to analyze consumption trends and adjust inventory levels, optimize costs by identifying the best suppliers, and reduce stock-outs through forecast-adjusted orders.
However, while machine learning is powerful, it doesn't guarantee 100 percent reliable inventory management over an entire year. It is based on past trends and struggles to anticipate exceptional events such as a pandemic, an economic crisis or geopolitical conflicts. A company also does not control supplier decisions, logistics delays or fluctuations in raw materials, all of which make fully automated planning difficult.
One of the assumptions of machine learning in inventory management is that it is enough to integrate delivery and manufacturing times to calculate optimal storage levels. However, this logic is regularly disrupted by unforeseen events such as strikes, supply difficulties for certain ingredients or data entry errors. Artificial intelligence, as advanced as it is, cannot anticipate these hazards and therefore requires human supervision. Contacts who are able to adapt to the unexpected and find alternative solutions are essential to ensure effective purchasing and inventory management.
Machine Learning as a decision-making tool
Machine learning has strong predictive potential, but its effectiveness depends entirely on how it is used. Contrary to the idea of a neutral and objective tool, it has the ability to adapt to its interlocutor, to the point of espousing their vision and expectations. This feature can be an asset when used by experts who are able to ask relevant questions and consider multiple scenarios. However, when used by someone who doesn't know how to structure their thinking, make assumptions, or question results, machine learning can become a counterproductive tool. Without a critical and methodical approach, it risks reinforcing pre-existing ideas rather than opening up reflection, thus locking the user into cognitive biases.
One of the major pitfalls of Machine Learning is the illusion of its neutrality. Many consider predictive models to be objective tools, whereas they are based on historical data that carries human biases. An AI that learns from past decisions can thus reproduce unintentional mistakes or discrimination. In addition, a static, unadjusted model quickly becomes obsolete and unsuited to new realities.
On the other hand, when used in a team, with well-constructed prompts to explore all possibilities and deepen the level of knowledge and precision, it becomes a real strategic ally. It is not a question of blindly relying on the results generated by AI, but of interacting intelligently with it, feeding it with various hypotheses and confronting its predictions with the reality on the ground. A collaborative approach transforms AI into a lever for collective intelligence, capable of revealing patterns and insights that might have escaped isolated human analysis.
Machine Learning is therefore a decision-making tool that can either be a factor in locking into pre-existing beliefs, or a great means of exploration and openness. It all depends on the rigor and intelligence with which it is used.
Machine learning has strong predictive potential, but its effectiveness depends on how it is used. He has the ability to adapt to his interlocutor, going so far as to espouse his vision and expectations. This feature can be an asset when used by experts who are able to ask relevant questions and formulate specific hypotheses. However, when used by someone who doesn't know how to structure their thinking or explore different avenues, machine learning can become a counterproductive tool. Without a critical and methodical approach, it risks producing biased results, reinforcing pre-existing ideas rather than opening up reflection.
On the other hand, when used in a team, with well-constructed prompts to explore all possibilities and deepen the level of knowledge and precision, it becomes a real strategic ally. It's not about blindly relying on the results generated by AI, but about interacting intelligently with it to refine analytics and adjust predictions based on the real-world context. It is this interplay between human expertise and algorithmic power that transforms machine learning into a truly effective tool for decision-making.
Conclusion
Machine learning is a powerful tool, but it's not a one-size-fits-all solution. While it excels in areas where data is structured and predictable, such as image recognition or weather forecasting, it shows its limits in environments where human decisions, emotion and intuition play a major role, such as sales management and purchasing.
The future of machine learning in the enterprise is therefore based on a hybrid approach, combining the analytical capabilities of algorithms with human expertise. AI must be a decision-making tool and not a substitute for professionals. It is by integrating this complementarity that companies will be able to maximize the impact of Machine Learning while minimizing its risks.
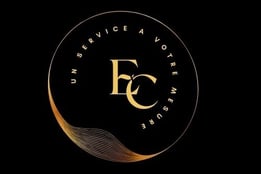
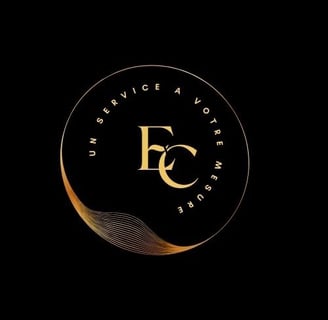
EUSKAL CONSEIL
9 rue Iguzki alde
64310 ST PEE SUR NIVELLE
FRANCE
0033782505766
euskalconseil@gmail.com
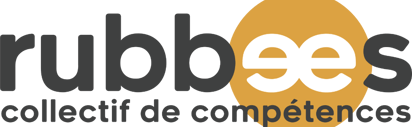
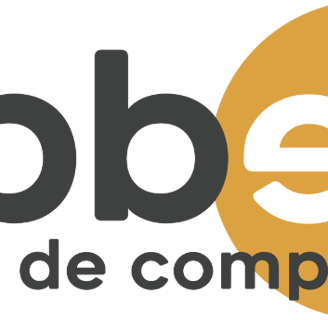